A Deep Dive into Using Algorithms to Detect Preferences from Brain Waves
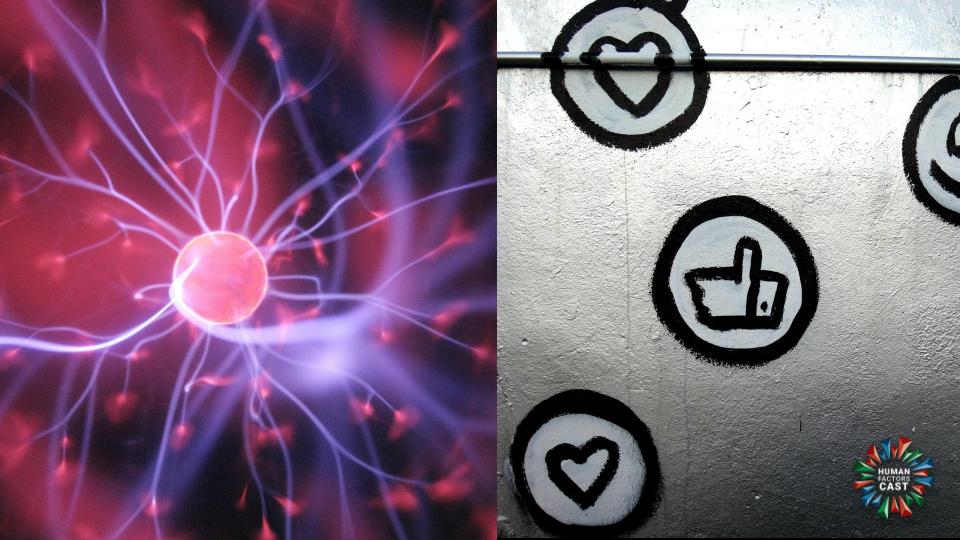
Listen to this blog post.
A Deep Dive into Using Algorithms to Detect Preferences from Brain Waves
It’s Wednesday again, and we know what that means: time for another deep dive! This week we will be diving into how we develop preferences, algorithmic bias, and a brief dip into what all of this might mean for our concept of free will.
How Do Our Preferences Form?
It is generally understood that different people have different preferences: one person might prefer chocolate ice cream, another might prefer vanilla. One person might prefer the cold, another might prefer the heat. The same person might prefer coffee in the morning and tea in the afternoon. What makes us have different preferences?
Daniel Bernoulli was a mathematician who, in 1738, developed our current understanding of how we make decisions: the expected utility hypothesis. The expected utility hypothesis can be simplified as we make decisions based on the value we expect to get from each choice by considering the probability of outcomes, our preferences, and the risk associated with each (humans are typically risk-averse).
A literature review in 2010 of preferences and values literature concluded that further research is needed to examine how goals, experience, and cognitive constraints influence preferences. It also encouraged researchers to continue developing contextually sensitive choice models. Together, these findings indicate that the context in which a decision is made can have a significant influence on our preferences. Similarly, a 2012 study in the International Journal of Game Theory suggested that our preferences are guided by what is “motivationally salient” to us at the time.
Further supporting the theory that context is an essential part of shaping our preferences is a study from 2015 that found that half of an individual’s facial preferences is unique to them, with variation accounted for by the significant impact that personal experiences have. When asked, the study’s author speculated that, based on prior research, greater exposure and more positive experience associations increase how attractive we find someone. This supports the idea that our preferences can change over time and are more influenced by how we individually experience the world than by our genes or the environment we grew up in.
Part of that context includes social factors. Economists have developed terminology for two types of preferences that come from external pressures: conspicuous consumption and information cascade. Conspicuous consumption is when we make choices (usually expensive ones) in order to build the image that we want to present to the world (e.g. We want to project that we are eco-friendly, so we drive an electric car, carry a reusable water bottle, and wear clothing made from sustainable fabrics). An information cascade is when so many other people are doing something, we go along with it (e.g. We may not need or particularly want a fidget spinner, but everyone is talking about them and buying them, so we buy one).
Racial Bias in Algorithms
Image source: Omar Lopez | Unsplash
Although we may not know exactly how they work, most of us are aware that much of our online experience is guided through algorithms that predict what we will be interested in. Social media algorithms suggest new videos to watch or people to follow, advertising algorithms suggest products to buy, search engines use a collection of algorithms to suggest results that will appeal to you - algorithms are in use everywhere.
Unfortunately, one aspect of algorithms that is still being figured out is how to get rid of racial bias. While algorithms are typically not purposefully designed to have a racial bias, oftentimes what seems like a small bit of programming results in larger racially discriminatory consequences.
A 2020 study in the New England Journal of Medicine found that:
- An algorithm developed by the American Heart Association to determine a heart failure risk score has led to more non-Black patients being referred to specialized care even when symptoms and medical history are the same
- An algorithm for a risk calculator used by thoracic surgeons predicts a higher risk of death and other complications from chest surgery for Black patients, leading to physicians being less likely to recommend them for life-saving procedures
- An algorithm that measures creatine as an indicator of kidney function adjusts creatine measurement levels in Black patients lower, potentially preventing them from receiving proper care
- An algorithm that is used for kidney transplants says that kidneys from Black kidney donors are more likely to fail, which ultimately leads to a reduced number of kidneys available to Black patients who need kidney transplants
- An algorithm that is used to determine if a mother needs to have a cesarean delivery because vaginal birth would be too dangerous applies a higher risk to women of color, setting them up for multiple cesarean deliveries (which is considered increasingly dangerous)
- An algorithm used by an online breast cancer screening tool calculates a lower risk for getting breast cancer for women of color even when all other risk factors are the same
Algorithmic bias is a huge problem in healthcare, and it is important to keep in mind that it extends beyond the healthcare setting, too. Racial bias in social media algorithms results in Black adolescents facing, on average, over five racial discrimination experiences per day. A computer that learned English by scraping the internet became racist and sexist.
An often-cited 2003 study found that White-sounding names received significantly more callbacks when applying for jobs than Black-sounding names. When this dataset was used to train an algorithm, it learned to automatically filter out the Black-sounding names.
In an article about racial bias in algorithms used for risk assessment in the judicial system, ProPublica explored how algorithms in use in the criminal justice system discriminate against Black defendants. In an analysis they performed on data from Broward County, Florida, ProPublica found that the COMPAS algorithm wrongly labeled Black defendants as future criminals at almost twice the rate of White defendants, and White defendants were mislabeled as low risk more often than Black defendants.
There is clearly a major, ongoing issue with racial bias in algorithms across all fields. This will need to be noted and addressed in any new algorithms to ensure they can benefit all potential end-users and not perpetuate harmful practices.
READ MORE |
If Our Preferences Can Be Predicted, Do We Have Free Will?
Image source: Karim MANJRA | Unsplash
Free will and whether or not we have it is a subject that truly deserves a deep dive all its own. While most of us have a general idea of what we think free will is - usually along the lines of our unique ability to be in control of our own actions, thoughts, and destiny - philosophers tend to disagree on a specific definition and method of measurement. There is also significant debate about if free will is simply an illusion.
Some in the psychological community take the position that our behaviors and thoughts are merely the collateral output of the physical happenings in the brain, the byproduct of neurons firing in reaction to stimuli, and mental processes have no influence over physical events. This is called epiphenomenalism.
Benjamin Libet's famous experiments seemed to prove epiphenomenalism, that we do not actually have free will because he found that our brain sent signals to start a behavior before we were aware of having decided to do the behavior. However, there is significant criticism of his findings. Al Mele and others have pointed out that Libet’s experiments did not typically look at events that were salient or important to an individual, the findings do not translate well to the real world, replicability of these findings is low or controversial, actions can be preceded by many things (e.g. urges, wants, intentions), we may plan an action for the future, and our intentions may be specific or relatively unspecific.
It seems that there is not enough data to say that we always have free will, but also not enough to say that we never have it.
What This Means for an Algorithm That Can Determine Our Preferences
A machine that can read our brain waves to determine what our preferences are could have many benefits, as we talked about on the show. It also may be seen as providing more support for the concept of epiphenomenalism: maybe our preferences are not the result of conscious thought, but are just the natural result of how our brains physically work.
If our preferences are so easy to determine by reading brain activity, are they really under our control? Do we really choose them, or is our body programmed to prefer one thing over another?
The idea that we do not have free will, that our actions are predetermined, can lead people to feel angry, decrease their desire to help others, and diminish any feelings of gratitude they may have. A device that puts the idea of free will into question may bring out these feelings in users.
Alternately, it may give users a feeling of control: if they know what their preferences are right now, they may be able to change them. After all, preferences change over time or given particular situations. It may also help users avoid choice paralysis (also called decision or analysis paralysis) by providing them with answers to certain choices they need to make throughout the day (e.g. Do I want a sandwich or a salad for lunch?).
Ultimately, it will be impossible to truly know how such a device might impact people until it is commercially available and adopted by a significant portion of the population. Still, it is fascinating to consider the potential implications.
The Human Factors Connection
Image source: ThisIsEngineering | Pexels
Designing products, services, and environments requires a certain amount of knowledge about user preferences: will users prefer certain labeling? Will users prefer a push button or a dial? As we have mentioned before, context will be crucial when taking into account user preferences.
While we can make adjustments to nudge users to prefer one option over another, a 2012 study found that when participants had the agency to make choices themselves they were much more likely to later say that their choice was the more desirable option than when a computer instructed the participants’ choices. The study also supported previous research that the act of choosing between two similar options can lead to enduring changes in preferences, with preference increasing for the option the participant chose.
These results indicate that by encouraging a user to repeatedly make decisions to choose a particular option, we can increase their preference for that option over time. When considering training programs or tasks that require repetitive action, we can keep this concept in mind as we apply psychology to our projects.
In a world of almost continuous technological advances, AI and machine learning are rapidly becoming commonplace. When working with machine learning and algorithms, it will be important to be mindful of potential racial bias. Some ways to mitigate bias in machine learning include:
- Matching the right model to the problem: there are many AI models available for use, so make sure that you take the time to consider which one is the best fit for what you are trying to do
- Use a representative training data set: try to get as close as you can to mirroring what the actual population looks like
- Monitor performance using real data: check your models and algorithms against real data as you develop them by using simple test questions
It is important to remember that bias is not restricted to the technical elements of machine learning: it is just as important to consider contextual factors as well. For example, the World Economic Forum’s 2018 Global Gender Gap Report found that only 22% of AI professionals are women. Black workers only account for 2.5% of Google’s entire workforce and 4% of Facebook’s and Microsoft’s. To address these issues and the bias they impart to AI, we can take action to:
- Remember that individual differences matter: pay attention to how diversity affects each part of the process and endeavor to get a number of different perspectives at each step to ensure you consider different points of view
- Recognize that diversity in leadership is essential for dealing with the underrepresentation of minority voices: a diverse leadership team will be more likely to encourage and amplify the contributions of team members who might not otherwise be acknowledged
- Make sure that there is accountability: organizations need to ensure they have a high level of diversity in their leadership and employees to minimize the chances that bias will creep into the project at any point in the process
Finally, we will need to keep in mind that how users will react to a device that reads brain waves to tell them (and likely others) their preferences is still largely unknown. However, we can help keep it positive by providing ways for users to use this information to their benefit. This may be by incorporating it into planning technology to help with decision paralysis, prompting users to support them in making changes if they want to, and make design choices with the user’s mental comfort in mind.
There is a lot we can take from considering algorithms that can determine our preferences by reading our brain waves. Much of it can be applied right now: the science of how our preferences are formed and can change and how we can decrease algorithmic bias, or at least notice it and adjust for it. We have some exciting human factors challenges ahead of us; it will be interesting to see how these technological advances impact our lives in big and small ways.
______________________________________________________________________________________________________
For more Human Factors Cast content, check back every Tuesday for our news roundups and join us on Twitch every Thursday at 4:30 PM PST our weekly podcast. If you haven't already, join us on Slack and Discord or on any of our social media communities (LinkedIn, Facebook, Twitter, Instagram).
Explore Some More
Episode Links
- Can Algorithms Detect Preferences from Brain Waves
- Human Factors Cast E210 - Can Algorithms Detect Preferences from Brain Waves?
- Original Article: Computers can now predict our preferences directly from our brain
How Do Our Preferences Form?
- Conspicuous consumption and the rising importance of experiential purchases
- Is He My Type? The Science Behind Mate Preference
- Preference Formation
- Preferences
- The Psychology of Preferences
Racial Bias in Algorithms
- Human Factors Cast E040 - I Smell Algorithmic Bias Afoot
- Human Factors Cast E111 - Gait Recognition, Bias in A.I., VR Railway Safety, MRI VR App
- Measuring Racial Discrimination in Algorithms
- When Things Go Viral: Youth’s Discrimination Exposure in the World of Social Media
- Why algorithms can be racist and sexist
If Our Preferences Can Be Predicted, Do We Have Free Will?
- Another Scientific Threat to Free Will?
- Breathing may change your mind about free will
- Free Will and Neuroscience
- How a Flawed Experiment “Proved” That Free Will Doesn’t Exist
- Libet and Free Will Revisited
- Philosophers and neuroscientists join forces to see whether science can solve the mystery of free will
- There’s No Such Thing as Free Will
The Human Factors Connection
- Algorithmic bias detection and mitigation: Best practices and policies to reduce consumer harms
- How to Beat ‘Analysis Paralysis’ and Make All the Decisions
- How to Make Changes in Life To Be the Best Version of You
- Wanted: Women in AI
Photo by HalGatewood.com from Unsplash (left)
Photo by George Pagan III from Unsplash (right)